I was recently hiking on a beautiful San Diego trail with two old friends.
Both are chemists. The first, a professional drug hunter deeply skeptical of AI. And the other a professor deeply interested in the field. As for myself, I’m a product manager for a molecular modeling and drug discovery AI.
As we walked, the professor asked a thought-provoking question:
Will AI alone be able to design a drug, and will this put us out of our jobs?
The skeptic replied:
Drug discovery AI will be like fusion energy – always twenty years away.
The lines seemed to be drawn. Fortunately we’re all scientists, and used to the process of challenging our perspectives. The only thing to do was to challenge our views with as much scientific rigor as an afternoon hike allowed.
As we walked and talked “into the weeds” we quickly began to realize that the answer to our prompt was far more nuanced. Confounding elements include the highly-protocolized nature of clinical trials, the art-like nuance of preclinical trials, and the unstructured nature of drug discovery itself.
In the end we all compromised – in light of the evidence – that artificial intelligence will expand human capabilities and give competitive advantages to drug hunters who apply the right AI solutions in the right context.
In short, AI won’t replace drug hunters. Drug hunters who use AI aptly will replace drug hunters who don’t.
In this article, I will endeavor to outline the evidence and arguments that led us to this conclusion. I should begin by outlining the perspective from which I am approaching this discussion.
By training, I'm a medicinal chemist that has developed clinical candidates (alongside my fair share of failed molecules). As a technology enthusiast I brought photoredox chemistry to Novartis, and built the most sophisticated automated lab in the world at Strateos.
.png)
My views on AI are neither optimistic nor pessimistic, they’re the views of a pragmatic scientist who – like many drug hunters – realizes that experiments are indifferent to our own emotions.
With that said, let’s dive into our thought experiment below.
Setting realistic expectations for AI
First – much as we did on our hike – we should come to a shared understanding of what AI is itself. What is it good at? What is it bad at? What’s a realistic present state? In a general sense, how could AI map to problems in drug discovery?
To begin, drug discovery is not a monolith. It’s an industry of nearly infinite complexity with many functions and requirements for many specialized intelligences. We can almost certainly do away with the possibility – at least at the present – that a single intelligence (artificial or human) could answer all drug discovery-related questions. This would require a “super intelligence”.
Despite the breakneck speed with which tools like LLMs are advancing in logic, planning, and general knowledge, we are a long way from a single intelligence that can provide expert-quality responses across the range of disciplines involved in drug discovery while still being grounded in specific facts of each.
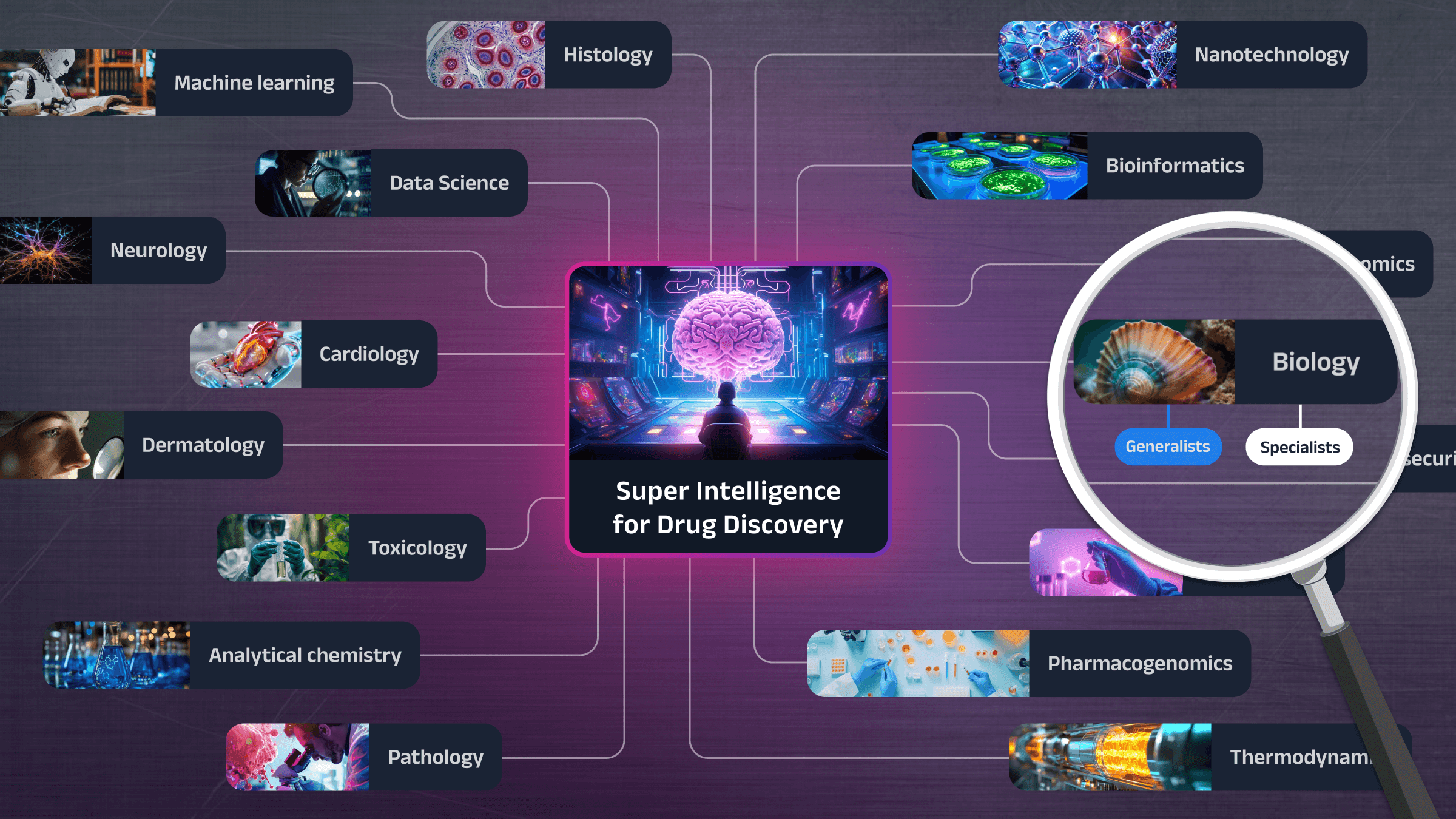
A more realistic approach is to build a balanced system across all relevant functional areas, and specialized intelligences for specific tasks. Perhaps such a system would qualify as a “super intelligence” – if you will – but only in the sense that current effective organizations based on human intelligence do already: systems that balance and augment generalists and specialists skillsets at scale.
Generative AI: how do cars work?
We all seemed to agree that in the present state, a balanced system of different intelligences (generalist and specialist) plugged into a large, human “super intelligence”-like organization is where there’s near-term opportunity. This reframes the original question to something like the following: what are the most impactful ways to augment human intelligence in drug discovery, rather than replace it?

To answer this question, let’s start by talking about what ideally happens at the interface of humans and AI.
In a general sense, AI is most useful as an algorithm that gives us answers. One growing subset of algorithms most closely linked to providing answers in simple terms is generative AI. It is perhaps the best suited of all AI to provide a connection between the operation of tools, manipulation of data, and the surrounding human organization. But it comes with an interesting challenge.
Generative AI is non-deterministic, meaning that the same input can generate slightly different outputs. This inherent uncertainty often requires adaptation by humans in a form of prompt engineering that is used to surface predictable behavior of common software. Fortunately, early experiences indicate that this transition comes naturally to people.
A second pressing issue for many generative AI uses is that systems can lack the factual foundation as well as human motivation to reliably work towards the real-world problems at hand. You have likely heard of “hallucinations”, the reason why ChatGPT provides the disclaimer “ChatGPT can make mistakes. Check important info.”
However, I need to caution the reader not to dismiss all generative AI engines because of hallucinations. These are just growing pains, not fundamental problems. One can compare the tendency to occasionally generate made-up stories to the behavior of a child.
.png)
For example, if I were to ask my young daughter “how do cars work” she would likely explain how they work in terms of fairies, pixies, and otherwise magical creatures. The point is not that she is incapable of describing the underlying mechanisms behind how cars work, it’s that she has not yet learned the type of answer one is looking for when they ask how cars work.
Similarly we can teach AI to recognize its own hallucinations as well as the types of answers we expect in a given context. Additionally, generative AI can be provided with tools to model the world accurately and in ways useful for scientific purposes. If my daughter had never heard of pixies or fairies, and instead had posters of internal combustion on her wall, she may well answer my query differently. As Garik Petrosyan, Deep Origin's Head of AI has mentioned for years "It's more effective to interact with non-deterministic AI as a person than a machine."
Simultaneously the non-deterministic nature of generative AI is an opportunity. It allows for greater flexibility of inputs, and the ability to generate novel insights – unexpected answers that allow humans to look at the problem from a new angle.
At Deep Origin, we’ve used these approaches as central elements to our design for Balto, the first AI assistant for drug discovery capable of operating world-class molecular modeling tools. With work Balto has shown significant progress in eliminating hallucinations and that access to the right tools – in this case molecular modeling tools – grounds answers and makes them useful in real world work. The ability to meet drug hunters where they are in natural language turns a much more flexible range of inputs into potential progress in the drug hunting process.
The many facets and problems of drug development
As I mentioned in the beginning, drug development is not a single, monolithic problem. It can be broken into stages with unique challenges including discovery (biology and chemistry), pre-clinical development, and clinical studies.

Clinical studies are the ultimate tests of a drug's potential, with many parameters and sprawling infrastructure. Acquisition of each data point is very expensive. Any improvement in a trial throughout or reduction in trial costs makes a large impact. This recent review summarizes specific aspects of clinical trials that are positively impacted by AI – including management of patient’s recruitment and dropout, clinical trial randomization and supply management, as well as extracting data from unstructured reports, data clean up and writing protocols.
Near term, I expect we will see additional applications around text processing with a gradual shift towards finding trends in multi-parameter data processing useful for better patient stratification or drug repurposing.
Prior to clinic, the preclinical state requires the art of balancing contradicting data and noisy correlations. In this stage, the active ingredient and formulation are fixed and the drug hunter needs to prove safety and to select the human dose.
It is important to emphasize that decisions made at this stage are existential to the careers of Chief Medical Officers and success of small to medium size biotechs. At this critical stage, AI starts to make an impact.
One noteworthy AI application generates a score for a clinical candidate that estimates overall success in the clinic. One can think of it as a molecular “credit score”. And a company can look at its portfolio of candidates and select ones that have the highest degree for success. I expect that the next generation of preclinical AI engines will be driven by deeper integration of population-based pharmacokinetic models (PBPK) and more scientific advances in translational biology.

Drug discovery: where AI can impact program trajectory the most
Drug discovery, where the drug development journey begins, can also benefit substantially from AI. This is the least structured and the most cinematic stage of drug discovery. This stage determines whether treatment is even theoretically possible. Improvements in speed and success rate here ripple out to the entire drug discovery enterprise, and have the potential to save innumerable lives, dollars, and hours.
While both biology and chemistry can be aided at the drug discovery stage, I would like to focus on AI for chemistry for now as my conversation on the hike was between chemists. AI for biology will be discussed in detail in the future blog. In chemistry, we can identify three general sub-stages where AI can help the most:
- Screening (finding seed molecules)
- Hit-to-lead (activity and selectivity optimization)
- And lead optimization (ADMET properties and PK/PD optimization)
Across these stages, AI has the ability to drive advances in one of the most common, essential, but exhausting tasks in science: summarizing and providing an interactive query of scientific literature. While this has shortcomings, it addresses a problem we all know but struggle to acknowledge: as humanity does more science, more papers are published, to the point where no single person can keep up with the explosion of publications (source, source). This is most acute in the early stages of drug discovery, where the cost of research is the lowest and publication highest. AI assistants, if appropriately trained to avoid hallucinations, can easily read and summarize papers, allowing researchers to do everything from extract key insights, understand papers in the broader context of literature, and act as a search tool for detailed information in supplementary information.
Beyond literature synthesis, generative AI engines recently gained the spotlight for hit-to-lead and lead-op stages. These models typically solve one of two important problems - identifying molecules in patentable chemical space or optimizing lead molecules towards the desired ADMET profile (adsorption, distribution, metabolism, excretion, and toxicity). Both approaches continue to evolve and to make an impact.
It’s worth noting, however, that there are two physical boundaries limiting success in these areas:
- Synthesizability - One can find that 30% of questions at “AI in Drug Discovery” conferences revolve around synthesizability and answers to these questions typically dance around “ready-to-make chemical libraries” or “talented CRO partner”.
- Quality of molecular simulation - Imprecise predictions increase the number of experiments required to validate hypotheses and, in the end, erode medicinal chemists' trust in theoretical predictions. While DiffDock made waves on publication, we and others have confidently found it to perform poorly, ranking below its open source non-AI predecessor AutoDock Vina
To bring forward the next generation of Generative AI engines, we need to eliminate these two boundaries. This is what we’ve been working on at Deep Origin where we’ve achieved higher prediction accuracy for docking and ultra large virtual screening.
Beyond better predictions, an emerging AI application that has potential to revolutionize the drug discovery process is the integration of AI chat interfaces with scientific tools.
While seemingly simple, it solves a problem that had no solution for over 30 years: the steep learning curve of molecular modeling tools.
Historically, these tools have been limited to relatively few experts because of the extensive knowledge required to evaluate simulation quality and experience needed to use the tools. Many simulation tools require months or years of training.
We’ve combined generative AI to promote accessibility to simulation tools alongside simulation quality itself in our product Balto, the first Drug Discovery AI Assistant.
Balto amalgamates our molecular modeling technologies with the ability to execute molecular modeling using natural language. The chat interface of Balto understands scientific context and guides users through simulation workflows, enabling a user of any experience to gather the necessary information about a particular protein target or a ligand and get state-of-the-art simulation results within the same portal. We hope this interaction between Human and Artificial Intelligences, coupled with continuous expansion and improvement of simulation tools, will improve the lives and work of researchers in drug discovery.
From watching scientists interact with Balto in closed beta, we think this is the missing piece to unlock the competitive advantages of AI and – as we mentioned in our section on “super intelligences” – a step towards unlocking the best of both human and artificial strengths.
The verdict
We left our hike after going deep in the weeds, past strong blanket statements about the future of work. As a pragmatist, I left even more excited about this era. And thanks to our meandering conversation I can’t help but bring up a few historical parallels.
Forty years ago, computers automated simple tasks and became essential to our life. Twenty years ago, smartphones made all these functions mobile and changed our habits again. Neither of these products were fully capable or featured in their first iterations. Today, among software claiming to streamline every aspect of our lives and work, we’re drowning in integrations, learning interfaces, and differently structured data. AI assistants build on all this to help us multiply the capabilities given to us in past technical eras and get back to the strategy and problem solving we all got into this field to pursue.
The examples I shared above show that AI is already making an impact in drug discovery. While skepticism remains, I suspect this era will be short and adoption rapid, because scientists are equipped to rationally evaluate – regardless of hype or skepticism. They will test, benchmark, and select the best tools for their critical work.
So how much talk of AI in drug discovery is hype? There’s some, but widespread opportunity and growing acceptance of real results.
I recommend everyone to pay attention to this field. You might be missing out, just like Nokia on buttonless interfaces.
AI will not be able to exceed capabilities of tools and algorithms that it relies on and there is nothing magical about it. Physical laws set boundaries for us all. But in a world where the ability to solve critical problems is gated by deep knowledge that is difficult to find and methods that require extensive training to use, AI offers the potential to shortcut and make us all productive, faster.