In this blog series including this and the upcoming posts we’ll attempt to cover the challenges in small molecule drug discovery, where computational approaches can help, and where they currently fall short.
One of the biggest challenges on the path to drug discovery remains ‘picking the right molecule’ – one that strikes an optimal balance between chemical properties, ensuring a safe and effective treatment for patients. This can be a daunting task, as the potential number of drug-like molecules far surpasses the total count of stars in the universe*. Identifying the ideal molecular candidate for drug development is essentially searching for a handful of structures within a pool of billions, and selecting the wrong one can easily lead to costly failures.
This is a known problem – the biotech landscape is littered with the graves of projects and companies who picked the wrong molecule and researchers have dedicated considerable resources to solving it. Some in vitro approaches, such as high-throughput screens that detect binding to a target protein, have been used for decades in drug discovery. But the breadth of the chemical space that can be tested experimentally is constrained by money, time, and synthesis limitations.1 Even popular methods like DNA-encoded libraries (DELs), capable of screening millions of compounds, cover relatively small regions of the chemical landscape and require physical synthesis of molecules in a world of limited human time and effort.2
In silico methods can overcome the limitations of in vitro assays, allowing computational evaluation of how molecules interact with the specific binding pocket of a target protein (Figure 1). These computational chemistry methods help researchers identify which molecules fit well, poorly, or not at all. In particular, docking and virtual screening enable researchers to test billions of molecules against a target at a fraction of the cost of an in vitro screen and without the bias arising from the difficulty of synthesis, making them a common starting point in drug discovery.
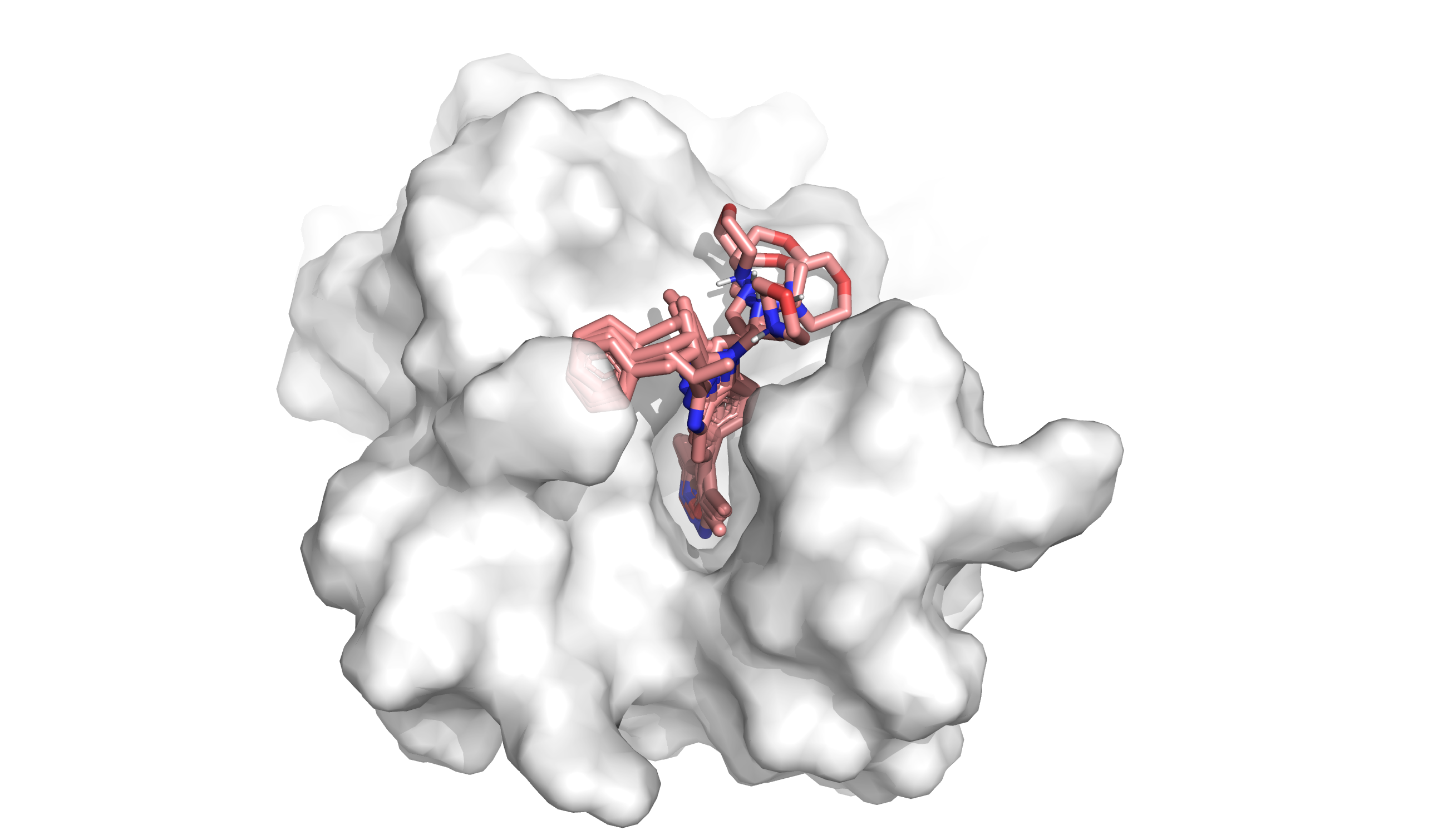
A quick primer on docking and virtual screening
In computational chemistry, docking interrogates how well individual molecules fit into and interact with part of a protein, called a ‘binding pocket’. Docking has three inputs: the protein structure, the ligand or small molecule structure, and the binding pocket location. It produces two outputs: the shape of the small molecule in the protein binding pocket (called a ‘binding pose’) and a score of how well the molecule binds (called a ‘docking score’). Researchers analyze the binding pose and docking score to understand how well a molecule fits and what to change about the molecule to improve its binding affinity.3 But docking analyzes a small number of molecules out of the 1060 possible small organic molecules in chemistry.4 Often we want to test the binding of billions (>109) of molecules at a time. How do we scale up?
This is where virtual screening comes in: methods optimized to test molecules efficiently at the scale of millions-to-billions of molecules (Figure 2). Virtual screening methods fall into two interconnected categories, structure-based virtual screening (SBVS) and ligand-based virtual screening (LBVS). SBVS includes techniques like scaled-up active learning docking and binding free energy calculations through molecular dynamics (MD) simulations and free energy perturbation (FEP). LBVS tools are pharmacophore, shape screening or talked-about generative de novo drug design methods.5 LBVS approaches also include machine learning-based quantitative structure-activity relationship (QSAR) methods, from Bayesian and tree-based techniques to cutting-edge neural networks-based methods. In contrast to SBVS, LBVS techniques don’t rely on the protein’s structure, but on the properties of other molecules that bind to the target – akin to finding a key that fits a lock by studying other keys, rather than the lock itself. Hope you're still with us and haven't been lost in the alphabet soup of abbreviations!

The beauty of virtual screening is its flexibility. Depending on the challenge at hand, we can use a single method or combine LBVS and SBVS techniques for a more comprehensive approach. If we have some clues about what works and what doesn’t for a target protein, we can start with LBVS methods. Or if we have no idea what would bind, we could start with comprehensive but computationally-intensive SBVS techniques. This can involve docking molecules to sift through billions of options down to a few promising ones. After that, we can tweak these molecules with some experiments and more targeted virtual screening, either structure-based or ligand-based, to boost their binding affinity. Here, we could also use computational binding affinity measurement methods through MD and FEP. These techniques help us understand how a molecule moves and how strongly it interacts with a binding pocket, ultimately helping us develop a better drug candidate.
And of course, binding affinity isn’t the only thing drug hunters care about. At the beginning, during the hit-finding phase, generally binding affinity is prioritized to discover those initial hit molecules that can bind to our target protein and get experimentally validated. But as the drug development moves forward, through stages like hit expansion, hit validation, hit-to-lead, and lead optimization, there's a growing focus on ADMET parameters. ADMET stands for administration, distribution, metabolism, elimination, and toxicity. These factors are crucial because they tell us how a drug behaves in the body, how safely it can be administered, and its overall effectiveness as a treatment. But, this is a topic for another blog post.
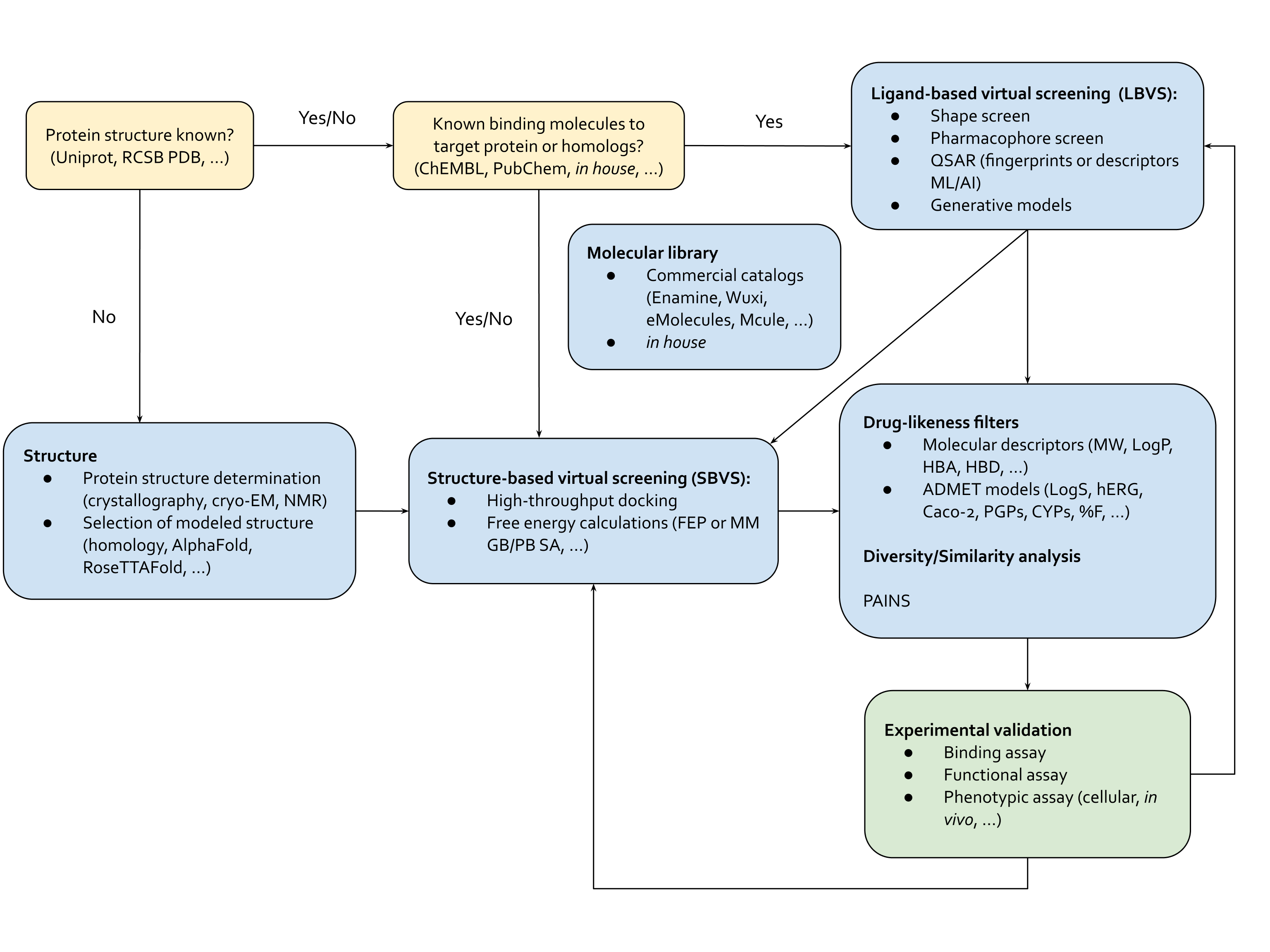
Why diverse billion compound libraries in virtual screening?
Diving into massive, diverse billion-compound libraries really changes the game for drug discovery, especially when we’re focusing on a specific protein target. Having such a huge pool of compounds at our disposal means we get tons of options for finding something that not only sticks to our target but does so in a cool, unique way. This wide selection allows us to pinpoint effective starting points and venture into novel regions of the vast chemical space unexplored for the given target protein6 .This potentially introduces fresh mechanisms of binding with new interesting interactions with the binding site of the target protein. Once an original hit is experimentally validated, large libraries are also more efficient at the hit expansion and analoging stage. Now we have the opportunity to zoom into the chemical region of the hit and explore a large number of analogs. Plus, starting with a big mix gives us better ways to fine-tune the drug's ADMET properties, which speeds up optimizing how the drug works and its safety in the body. This approach also means we’re not back to square one if a compound turns out less than ideal; instead, we've got other promising ones to keep moving forward with, saving us time down the line.
And finally…
So, after we've got a handle on which virtual screening method to use and why virtual screening, especially applied to diverse and large compound libraries, is important, we can now quickly return to docking. Docking is where we get down to brass tacks. It's like trying to fit puzzle pieces together – seeing how small molecules snug up into the protein's binding site. This step is crucial because it shows us what might actually work when we move from computer screens to the real world of drug discovery. Docking doesn't just help us pick out the promising candidates from the virtual crowd; it also gives us a sneak peek at how these molecules might behave in real life. It's a key player in the game, guiding us from theory to practice as we hunt for new drugs.
Docking and virtual screening have been rock stars of drug discovery for over 30 years. Think AutoDock Vina, Glide, or the buzz-worthy, neural-net based DiffDock – these tools are like the unsung heroes in the epic quest for new drugs. They start the show, turning a sea of billions of possibilities into a VIP list of around 100 molecules, ready for the in vitro spotlight. They're not just narrowing down the list; they're giving us the insider tips on how to tweak these molecules to make them hit just right.
At Deep Origin we developed a state-of-the-art docking and incorporated active learning techniques to efficiently screen billion compound libraries. In benchmarking our docking significantly outperforms other methods like DiffDock, Smina, particularly in ligand ranking and pose prediction accuracy. With increased accuracy and speed of docking we can identify potential drug leads easier. Here is more information about our docking capabilities.
But hold your applause – it’s not all smooth sailing. Even these rock stars have their pitfalls. They're not always the magic bullet in our quest for blockbuster drugs. In our next piece, we'll dive into the nitty-gritty of what's tripping up docking and virtual screening. We're talking about the hurdles, the slip-ups, and why, sometimes, they fall short in stopping drug development from hitting sour notes. Stay tuned as we uncover the other side of these drug discovery headliners.
Keen to learn more? Join us on May 2nd, 12PM PT for our introduction to computational drug discovery webinar!
Footnotes:
* There are approximately 10^24 stars in the universe, and 10^60 possible druglike small molecules.5, 7
References:
- Lyu, J., Wang, S., Balius, T.E. et al. Ultra-large library docking for discovering new chemotypes. Nature 566, 224–229 (2019). https://doi.org/10.1038/s41586-019-0917-9
- Kenny, S.E.; Antaw, F.; Locke, W.J.; Howard, C.B.; Korbie, D.; Trau, M. Next-Generation Molecular Discovery: From Bottom-Up In Vivo and In Vitro Approaches to In Silico Top-Down Approaches for Therapeutics Neogenesis. Life 2022, 12, 363 (2022). doi: 10.3390/life12030363
- Peterson, A.A., Liu, D.R. Small-molecule discovery through DNA-encoded libraries. Nat Rev Drug Discov 22, 699–722 (2023). https://doi.org/10.1038/s41573-023-00713-6
- Kitchen, D., Decornez, H., Furr, J. et al. Docking and scoring in virtual screening for drug discovery: methods and applications. Nat Rev Drug Discov 3, 935–949 (2004). https://doi.org/10.1038/nrd1549
- Bohacek, R.S., McMartin, C. and Guida, W.C. (1996), The art and practice of structure-based drug design: A molecular modeling perspective. Med. Res. Rev., 16: 3-50. https://doi.org/10.1002/(SICI)1098-1128(199601)16:1<3::AID-MED1>3.0.CO;2-6
- Vázquez J, López M, Gibert E, Herrero E, Luque FJ. Merging Ligand-Based and Structure-Based Methods in Drug Discovery: An Overview of Combined Virtual Screening Approaches. Molecules. 2020 Oct 15;25(20):4723. doi: 10.3390/molecules25204723. PMID: 33076254; PMCID: PMC7587536.
- Reymond JL. The chemical space project. Acc Chem Res. 2015 Mar 17;48(3):722-30. doi: 10.1021/ar500432k. Epub 2015 Feb 17. PMID: 25687211